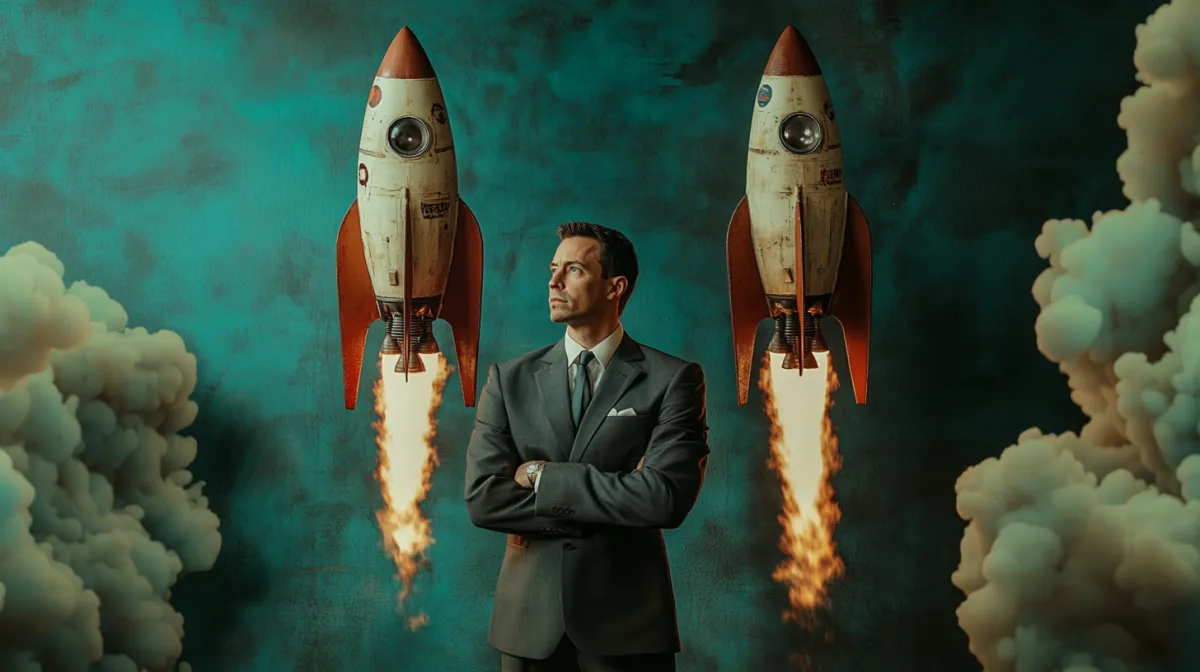
Deeper Insights for Business Success with Advanced Analytics Techniques
Any business hoping to stay competitive in the data-driven world of today needs to be able to extract valuable insights from data. Advanced analytics methods offer valuable tools for identifying patterns, predicting trends, and making informed decisions. This guide explores advanced analytics methods, their applications, and the potential advantages they offer to businesses.
Understanding Advanced Analytics
Advanced analytics is the name given to a collection of techniques and procedures used to examine complex data sets in order to find deeper insights that conventional analytics could miss. Among other functions, it offers machine learning, data mining, and predictive analytics. Advanced analytics tries to offer a deeper knowledge and prediction power than basic analytics, which is usually concerned with descriptive data and reporting.
Relevance of Advanced Analytics
1. Informed Decision-Making: Companies may make better strategic decisions when advanced analytics gives them useful insights.
2. Competitive Advantage: Businesses that apply advanced analytics can significantly outperform their competitors by comprehending consumer behaviour and market trends.
3. Efficiency & Cost Savings: By identifying inefficiencies and simplifying processes, advanced analytics can drastically cut expenses. Advanced analytics help businesses stay ahead of industry trends and find new opportunities that promote creativity.
Principal Advanced Analytics Methodologies
A. Analytics that predict.
Using historical data, statistical techniques, and machine learning techniques, predictive analytics projects future outcomes. This method helps businesses to foresee trends and events, which facilitates more proactive decision-making.
Applications of Predictive Analytics
1. Retailers: Retailers employ predictive analytics to predict consumer purchases and customize marketing initiatives.
2. Risk Management: Skilled risk management and improved loan default prediction are capabilities of financial institutions.
3. Supply Chain Optimization: In order to lower waste and increase efficiency, businesses ascertain demand and adjust inventory levels.
Methodologies for Analytics Predictive.
Regression analysis is a technique that models the relationship between variables to forecast results.
• Time Series Analysis: Estimate future trends by using data points gathered or documented at exact time intervals.
• Machine Learning Algorithms: Decision trees, neural networks, and support vector machines are a few examples of machine learning algorithms that make data-driven predictions.
B. Robotic.
Within artificial intelligence (AI), machine learning teaches algorithms to identify trends in data and make judgments without explicit programming. More data exposure leads to machine learning models getting better over time.
Different Machine Learning Types
1. Supervised Learning: The model is trained with labelled data, hence the outcome is already known. Among the most often used algorithms are logistic regression, support vector machines, and linear regression.
2. Unsupervised Learning: Through training on unlabeled data, the model is able to recognize patterns or groupings. Some often used techniques are clustering and association.
3. Reinforcement Learning: By means of contact with its environment and feedback in the form of rewards or penalties, the model learns.
Relevance of Machine Learning
• Fraud Detection: Using machine learning, financial services are able to detect fraud in real-time.
• Identify Patterns: Marketers classify consumers according to their buying patterns using clustering algorithms.
• Predictive Maintenance: Reduce downtime and maintenance expenses by manufacturers foreseeing equipment problems.
C. Data Mining.
Data mining is the study of patterns, correlations, and anomalies in enormous volumes of data. Statistical analysis, machine learning, and database systems all extract useful information.
Methodologies of Data mining
1. Assigns objects from a collection to particular classes or categories. Emails, for instance, might be categorized as spam or not.
2. Clustering: The procedure of classifying a collection of items such that items within the same group are more similar than those within others. Look at customer segmentation, for instance.
3. Association Rule Learning: Finds fascinating correlations between variables in huge databases. Market basket analysis, for instance, shows what is often bought together.
Applications of Data Mining
• Analysis of the Market Basket: Retailers use association rules to determine trends of product purchases.
• Customer Churn Analysis: Telecommunications firms create retention plans and assess the reasons of customer churn.
• Healthcare: Identifying trends in patient data to forecast disease outbreaks and enhance available treatments.
NLP and Text Analytics
Text analytics and Natural Language Processing (NLP) are the fields of text data extraction for relevant information. This includes extracting context, attitude, and patterns from unstructured data sources as customer reviews, social media posts, and so on.
Methods in natural language processing
1. Sentiment analysis: Establishes the positive, negative, or neutral sentiment conveyed in text.
2. Subject Modelling: Determines the subjects included in a collection of papers.
3. Named Entity Recognition (NER): it takes names, organizations, and places out of text.
Text analytics and natural language processing applications
Businesses use social media sentiment analysis as a tool to keep an eye on public opinion and manage the reputation of their brands.
• Analyzing Customer input: Companies use consumer input to get understanding of how to enhance their offerings.
• Chatbots and Virtual Assistants: NLP allows smart chatbots to understand and respond to customer questions in natural language.
Analytical Big Data
Big data analytics is the methodical study and information extraction from data sets too big or complex for conventional data processing software. Utilizing sophisticated algorithms and parallel computing, it handles enormous volumes of data.
Methodologies in Analytics of Big Data
1. Hadoop: The Hadoop Ecosystem is a platform for widely dispersed computer cluster processing of huge data collections.
3. Spark: Spark is a fault-tolerant and implicitly data parallel distributed computing technology that offers an interface for programming entire clusters.
2. NoSQL Databases: These are made to handle enormous volumes of unstructured data and offer scalability and flexible schema design.
Applications of Analysis of Big Data
• Consumer Insights: To comprehend the interests and behaviours of their customers, retailers analyze enormous volumes of transaction data.
• Predictive Analytics: Financial institutions use market data to forecast stock movements and guide investment decisions.
• Operational Effectiveness: Manufacturing companies use equipment sensor data to enhance production procedures and lower downtime.
Using Advanced Analytics in Commercial Environments
Application of Advanced Analytics
1. State Goals: Make it very evident what you want to accomplish with sophisticated analytics.
2. Gather Pertinent Data: Sustain consistency and quality of the data.
3. Choose the Correct Tools and Technologies: Depending on your objectives and the type of your data, choose the appropriate tools and technologies.
4. Organize a Competent Group: Put together a group of domain experts, analysts, and data scientists.
5. Build and Verify Models: Using past data, build and verify predicted models.
6. Deploy and Monitor: Put the models in practical settings and keep an eye on their activities all the time.
Implications of Advanced Analytics
1. Data Quality: Before starting any study, it is imperative that the data is correct and comprehensive.
2. Talent Gap: There is usually more of a need than supply for qualified data scientists and analysts.
3. Integration: It can be difficult to include cutting-edge analytics technologies into current systems.
4. Cost: For small companies in particular, implementing sophisticated analytics systems can be expensive.
5. Change Management: In order to ensure staff buy-in and appropriate application of analytics insights, organizations must manage change successfully.
Good Practices
1. Start Small: Start modest and use test projects to show off the benefits of advanced analytics.
2. Emphasize High-Impact Areas: Give top priority to those where analytics may add the most value.
3. Develop a Culture of Data Driven Appreciation: Promote an organization-wide culture that values data and analytics.
4. Ongoing Education: Stay current with analytics methods and tools.
5. Collaboration: Promote cooperation amongst domain experts and data scientists to improve the precision and applicability of analytics results.
Trends to Come in Advanced Analytics.
A. Machine learning & AI
More complex models providing deeper insights and automation opportunities are predicted to drive the continued integration of AI and machine learning into advanced analytics.
B. Augmented Analytics
Augmented analytics uses AI to enhance data preparation, insight creation, and data visualization, therefore opening up analytics to non-experts.
C. Edge analytics
Real-time insights and actions will become possible as the number of Internet of Things devices increases and edge analytics—processing data closer to the source rather than in a central data center—becomes more widespread.
D. Artificial Intelligence That Explains
Explainable AI aims to make complex machine learning algorithms more understandable and transparent for humans, hence boosting responsibility and confidence.
E. Privacy of Data and Ethics.
Stronger data governance structures will be needed as data collection and analytics activities proliferate, and ethical and privacy concerns grow.
Conclusion
Modern analytics techniques are completely changing the way that companies operate and offer hitherto unheard-of opportunities for development, optimization, and insight. Companies that grasp and use these strategies can reach previously unheard-of heights of productivity and creativity. But effective execution requires careful preparation, qualified staff, and a commitment to fostering a culture that is data driven. Maintaining a competitive edge in the market will require being up-to-date on the latest trends and best practices in advanced analytics as technology develops.
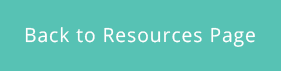